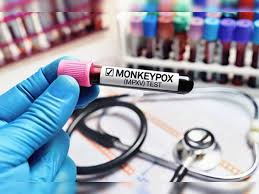
The outbreak of Monkeypox in 2022 presented a significant public health challenge worldwide. However, the narrative that quickly emerged—suggesting that Monkeypox primarily affected homosexual men—led to stigma, misinformation, and a misdirected public health response. This misunderstanding was largely fueled by the misinterpretation of statistical data, which highlighted the urgent need for more nuanced analytical approaches. At Bogoni Research, we believe that Bayesian statistics could have played a crucial role in preventing these errors, ensuring that public health messaging was both accurate and effective.
The Problem: Misleading Statistical Interpretations
As Monkeypox cases began to rise, early reports focused on clusters of cases among men who have sex with men (MSM). While these reports were statistically accurate in describing the initial data, they failed to account for the broader context and the dynamic nature of the outbreak. The data was presented in a way that suggested a strong association between Monkeypox and homosexual behavior, which led to public health policies and media coverage that inadvertently stigmatized an entire community.
This misinterpretation occurred due to several key factors:
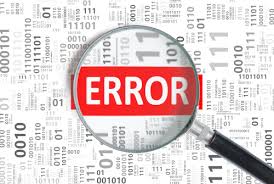
Overreliance on Initial Data:
The early cases were predominantly reported in MSM communities, but this was likely due to increased testing and reporting within these groups rather than a true epidemiological link.
Lack of Contextualization:
The data was not adequately contextualized with broader epidemiological factors, such as contact tracing, behavior patterns, and public health outreach efforts that focused on specific communities.


Static Analysis:
Traditional frequentist approaches provided a snapshot of the outbreak at a given time, failing to account for how the situation might evolve as more data became available.
Our Approach: Leveraging Bayesian Statistics for Accurate Public Health Reporting
At Bogoni Research, we propose a different approach—one that could have provided a more accurate and less harmful narrative. By employing Bayesian statistics, public health authorities could have continually updated their understanding of the outbreak as new data emerged, reducing the likelihood of premature conclusions and ensuring a more balanced public health response.
Here’s how Bayesian analysis could have made a difference:
- Incorporating Prior Knowledge: Bayesian statistics allows for the integration of prior knowledge into the analysis. For example, historical data on previous Monkeypox outbreaks, which were not linked to sexual behavior, could have been used as a prior to temper the initial data. This would have prevented the early reports from disproportionately focusing on MSM, offering a broader perspective from the outset.
- Dynamic Data Updating: As the outbreak progressed and more data became available from different populations, the Bayesian model could have continuously updated the probability of different transmission routes. This dynamic updating would have provided a more accurate picture of the outbreak, reducing the risk of stigmatization and ensuring that resources were directed where they were truly needed.
- Balancing Uncertainty: Bayesian analysis provides a full probability distribution rather than a single point estimate, offering a more nuanced understanding of the uncertainty involved in early-stage data. This would have allowed public health officials to communicate the evolving nature of the outbreak more effectively, emphasizing that the initial data was not definitive.
- Informing Public Health Strategies: With Bayesian models, public health authorities could have better identified high-risk groups based on updated evidence, ensuring that interventions were both effective and equitable. This would have helped avoid the harmful focus on MSM and instead directed efforts towards broader at-risk populations.
The Potential Impact: Reducing Stigma and Improving Public Health Outcomes
Had Bayesian statistics been employed during the Monkeypox outbreak, the narrative could have been significantly different. Rather than focusing on a specific group, public health messaging would have reflected the complexity of the situation, acknowledging the uncertainty and evolving nature of the outbreak. This approach would have likely reduced the stigma associated with the disease, leading to more effective and inclusive public health interventions.
How Bogoni Research Can Help
At Bogoni Research, we specialize in applying advanced statistical methods like Bayesian analysis to complex public health challenges. We understand the importance of accurate data interpretation and the need for dynamic models that evolve with the situation. Whether it’s in the midst of a health crisis or during routine epidemiological surveillance, our team is equipped to provide the insights needed to avoid missteps like those seen in the Monkeypox outbreak.
We offer:
- Custom Bayesian Models: Tailored to specific public health needs, allowing for real-time updates and more accurate predictions.
- Expert Data Interpretation: Helping public health authorities understand the full context of the data, ensuring balanced and effective messaging.
- Strategic Consultation: Guiding decision-makers on how to best use statistical insights to shape public health strategies and communication.
With the right approach, we can prevent the spread of misinformation, reduce stigma, and save lives. Let’s ensure that public health data works for everyone—not just the headlines!