Unraveling Statistical Metrics: Understanding Coefficient of Variation, Root MSE, and R-squared
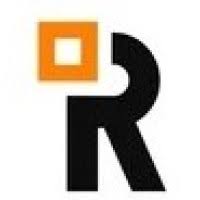
In the realm of statistical analysis, researchers are often confronted with a plethora of metrics that serve as guiding lights in the interpretation of data and assessment of model reliability. Among these metrics, Coefficient of Variation (CV), Root Mean Square Error (Root MSE), and R-squared (R²) stand out as essential tools, offering valuable insights into variability, predictive accuracy, and explanatory power. Coefficient of Variation (CV) measures the relative variability of data points, expressing the standard deviation as a percentage of the mean. In a SAS output, CV is presented as a percentage, reflecting the dispersion of data around the mean and signaling potential issues such as heteroscedasticity.
Root Mean Square Error (Root MSE) serves as a benchmark for predictive accuracy, quantifying the average deviation of observed values from predicted values in a regression model. Lower Root MSE values in SAS output indicate better model fit and predictive accuracy, while higher values may suggest the need for model refinement.
R-squared (R²) is a measure of the proportion of variance in the dependent variable explained by independent variables in a regression model. Ranging from 0 to 1, R-squared values in SAS output provide insights into the explanatory power of the model, with higher values indicating better fit and greater predictive capability. Understanding the interpretation of these metrics in a SAS output empowers researchers to assess the reliability and validity of their analyses, guiding them toward more informed decisions and meaningful interpretations of data.